Abstract:
Cancer is an evolutionary process characterised by profound intratumoral heterogeneity. From the genome, intratumoral heterogeneity can be quantified using in silco estimations of cancer cell fractions of tumour-specific somatic mutations. This estimation problem fits squarely in the area of mixture models. In the first part of the talk, I will present two closely related mixture models that can effectively estimate cancer cell fractions of point mutations and structural variants. Using large cohorts of whole exome and genome profiles across multiple cancer types, cancer cell fraction estimates reveal interesting patterns of evolutionary dynamics underlying cancer evolution.
In the second part, I will showcase how unsupervised representation learning models can improve quantification of heterogeneity within histological images of tumour slides. These representation learning models include deep generative models and self-supervised models. Using images across Breast, Colon and Lung tumours, these models capture distinct phenotypic characteristics of tissue samples, including cancer cell destiny, tissue types and histological subtypes, paving the way for further understanding of tumor progression and tumor micro-environment, and ultimately refining histopathological classification for diagnosis and treatment.
About the Speaker:
Ke Yuan is a Lecturer (Assistant Professor) in Machine Learning and Computational Biology at the School of Computing Science and the Institute of Cancer Sciences, University of Glasgow. His main research interest is developing novel machine learning and statistical methods for genomic and image data to answer questions in cancer and virus evolution. Prior to Glasgow, he received a PhD from the University of Southampton in 2013 advised by Prof Mahesan Niranjan. Till 04/2016, he was a postdoctoral research fellow at Cancer Research UK Cambridge Institute at the University of Cambridge working with Dr Florian Markowetz.
Tecent Meeting: https://meeting.tencent.com/s/wQMLOqpT3bfc
Meeting ID:662 202 344
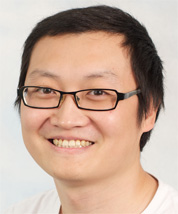