Abstract:
Predicting future outcome values based on their observed features using a model estimated on a training data set in a common machine learning problem. Many learning algorithms have been proposed and shown to be successful when the test data and training data come from the same distribution. However, the best-performing models for a given distribution of training data typically exploit subtle statistical relationships among features, making them potentially more prone to prediction error when applied to test data whose distribution differs from that in training data. How to develop learning models that are stable and robust to shifts in data is of paramount importance for both academic research and real applications. Causal inference, which refers to the process of drawing a conclusion about a causal connection based on the conditions of the occurrence of an effect, is a powerful statistical modeling tool for explanatory and stable learning. In this talk, we focus on the latest progress of stable learning, aiming to explore causal knowledge from observational data to improve the interpretability and stability of machine learning algorithms.
Biography:
Peng Cui is an Associate Professor with tenure in Tsinghua University. He got his PhD degree from Tsinghua University in 2010. His research interests include causal inference and stable learning, network representation learning, and social dynamics modeling. He has published more than 100 papers in prestigious conferences and journals in machine learning, data mining and multimedia. His recent research won 5 best paper awards and were selected into the Best of KDD special issues in 2014 and 2016 respectively. He is the Associate Editor of IEEE TKDE, IEEE TBD, ACM TIST, ACM TOMM, DMKD and KAIS etc., and the program co-chair of ACM CIKM19 and MMM2020. He is a Distinguished Member of ACM and CCF, and Senior Member of IEEE.
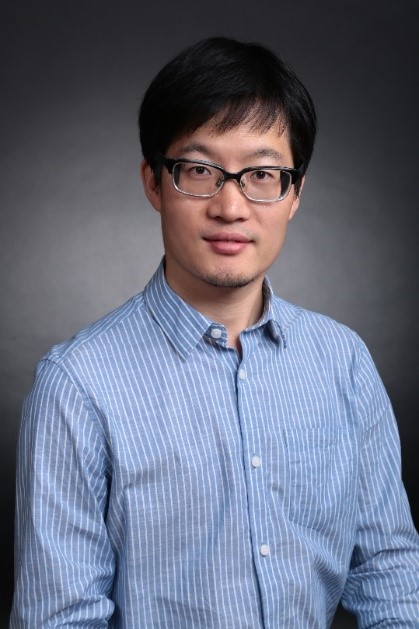
Tencent Meeting:https://meeting.tencent.com/dm/wwY5cu6UWaLD
Meeting ID:730 557 727