报告人:Fanghui Liu(EPFL, Switzerland)
时间:2023-09-11 10:30-11:30
地点:Room 1513, Sciences Building No. 1
Abstract:
The conventional wisdom of simple models in signal processing and machine learning misses the bigger picture, especially over-parameterized neural networks (NNs), where the number of parameters are much larger than the number of training data. Our goal is to explore the mystery behind over-parameterized NNs from a theoretical side.
In this talk, I will discuss the role of over-parameterization in neural networks, to theoretically understand why they can perform well in terms of benign overfitting and double descent. Furthermore, I will talk about the robustness of neural networks, affected by architecture and initialization in a function space theory view. It aims to answer a fundamental question: over-parameterization in NNs helps or hurts robustness?
Brief bio:
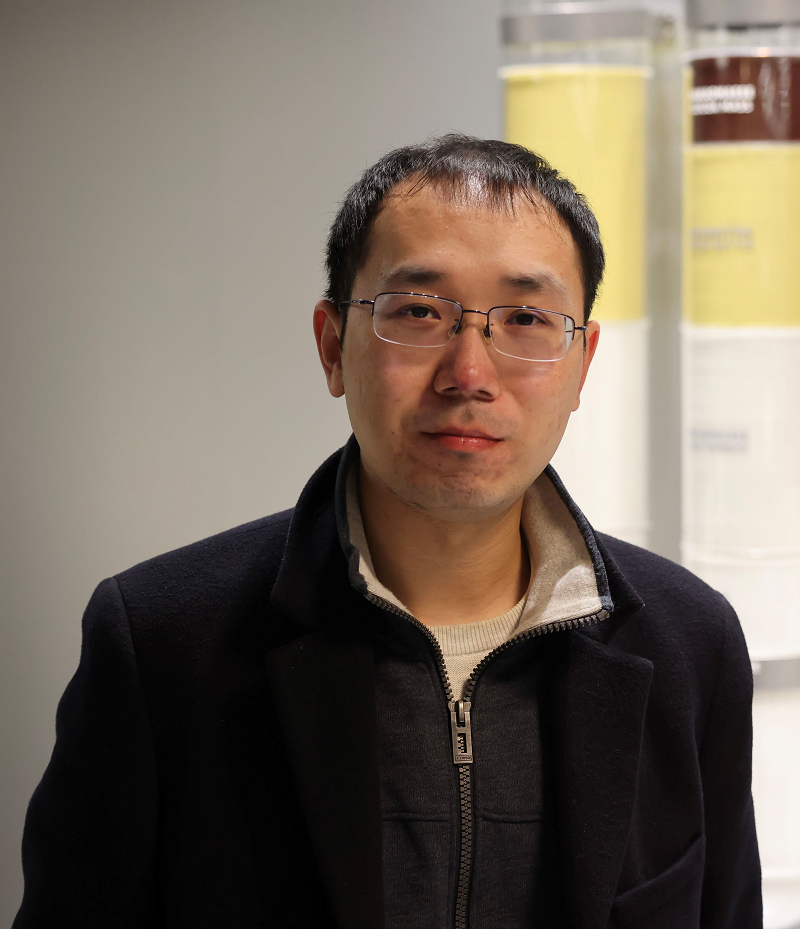
Fanghui Liu is currently a postdoctoral researcher at EPFL, Switzerland and will be an assistant professor at University of Warwick, UK next month. His research interests focus on statistical learning theory, to build the mathematical foundations of machine learning. For his work on learning theory and cooperation, he was chosen for Rising Star in AI (KAUST 2023) and presented two tutorials at ICASSP 2023 and CVPR 2023. Prior to his current position, Fanghui received his PhD from Shanghai Jiao Tong University and worked as a postdoc researcher at KU Leuven, Belgium.