Simultaneous inference for monotone and smoothly time-varying functions under complex temporal dynamics
报告人:吴未迟 (清华大学)
时间:2024-9-26 14:00-15:00
地点:智华楼盈不足-109
Abstract:
We propose a new framework for the simultaneous inference of monotone and smoothly time-varying functions under complex temporal dynamics. This will be done utilizing the monotone rearrangement and the nonparametric estimation. We capitalize the Gaussian approximation for the nonparametric monotone estimator and construct the asymptotically correct simultaneous confidence bands (SCBs) using designed bootstrap methods. We investigate two general and practical scenarios. The first is the simultaneous inference of monotone smooth trends from moderately high-dimensional time series. The proposed algorithm has been employed for the joint inference of temperature curves from multiple areas. Specifically, most existing methods are designed for a single monotone smooth trend. In such cases, our proposed SCB empirically exhibits the narrowest width among existing approaches while maintaining confidence levels. It has also been used for testing several hypotheses tailored to global warming. The second scenario involves simultaneous inference of monotone and smoothly time-varying regression coefficients in time-varying coefficient linear models. The proposed algorithm has been utilized for testing the impact of sunshine duration on temperature which is believed to be increasing due to severe greenhouse effect. The validity of the proposed methods has been justified in theory as well as by extensive simulations.
Bio:
吴未迟,清华大学统计与数据科学系副教授。主要研究方向为复杂时间序列,非参数统计,统计网络,变点问题。在Annals of Statistics, Biometrika, Journal of Business and Economic Statistics, Bernoulli 等期刊上发表多篇文章。担任Statistics and Probability Letters 的Associate Editor。
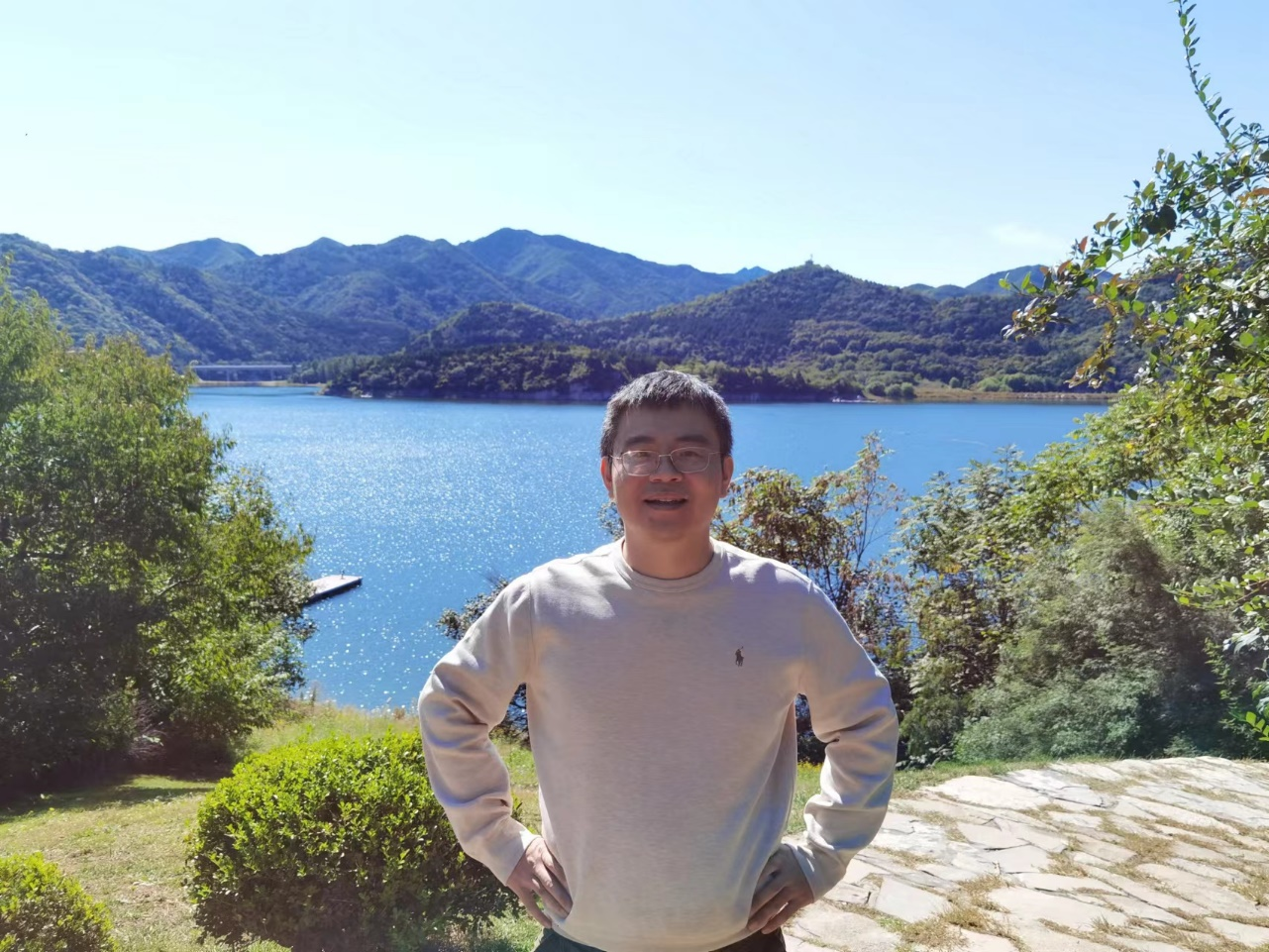