Debiased calibration estimation using generalized entropy under selection bias
报告人:Jae-kwang Kim(Iowa State University)
时间:2024-10-17 14:00-15:00
地点:智华楼四元厅-225
Abstract:Incorporating the auxiliary information into the survey estimation is a fundamental problem in survey sampling. Calibration weighting is a popular tool for incorporating the auxiliary information. The calibration weighting method of Deville and Sarndal (1992) uses a distance measure between the design weights and the final weights to solve the optimization problem with calibration constraints.
In this paper, we propose a new framework using generalized entropy as the objective function for optimization. Design weights are used in the constraints, rather than in the objective function, to achieve design consistency. The new calibration framework is attractive as it is general and can produce more efficient calibration weights than the classical calibration weights. Furthermore, we identify the optimal choice of the generalized entropy function that achieves the minimum variance among the different choices of the generalized entropy function under the same constraints. Asymptotic properties, such as design consistency and asymptotic normality, are presented rigorously. An extension of the proposed method to doubly robust propensity score estimation will also be presented.
Bio
Jae-kwang Kim is a professor in Statistics department at Iowa State University (ISU) . He is a fellow of ASA and IMS and named to a Liberal Arts and Sciences Dean's Professor from the college of Liberal Arts and Sciences at Iowa State University. His main research interest lies in survey sampling and statistical analysis with missing data, and related topics in measurement error, multi-level models, and data integration. His recent work focuses on machine learning topics such as function estimation under reproducing kernel Hilbert space.
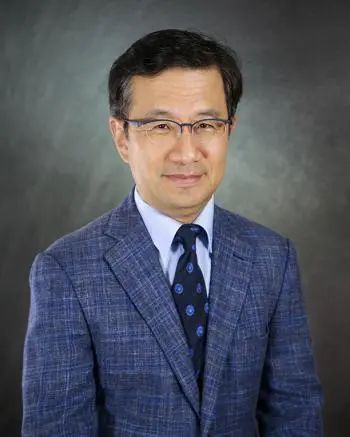